Introduction to Photonic Integrated Circuits
Photonic Integrated Circuits (PICs) represent a groundbreaking shift in how we approach data transmission, processing, and sensing. By harnessing the power of light, these circuits offer a promising pathway towards faster, more efficient communication systems. Unlike traditional electronic ICs that rely on electrons, PICs use photons for signal processing, leading to minimal loss and cross-talk, even over vast distances. This capability is crucial in today's data-driven world, where the demand for bandwidth and processing speed is ever-increasing.
PICs are not just limited to improving communication technologies; they also open new avenues in a variety of fields such as quantum computing, medical diagnostics, and environmental sensing. The ability to integrate multiple optical components onto a single chip means that PICs can perform complex operations more efficiently and at a smaller scale than ever before.
The development of deep photonic network platforms, as detailed in the document, marks a significant advancement in this field. By enabling the design of optical devices with arbitrary and broadband functionality, such platforms pave the way for the next generation of PICs, offering unprecedented flexibility and performance. This introduction to photonic integrated circuits sets the stage for exploring the innovative deep photonic network architecture and its potential to revolutionize optical systems across various applications.
Deep Photonic Network Architecture
The architecture of deep photonic networks represents a leap forward in the design and functionality of photonic integrated circuits. At its core, this architecture utilizes Mach-Zehnder Interferometers (MZIs), which are configurable optical elements capable of manipulating light in precise and complex ways. This modular design approach allows for the construction of large-scale optical networks on a chip, capable of performing a wide range of functions from signal processing to complex computational tasks.
Key to the deep photonic network's design is its scalability and flexibility. By arranging MZIs in a deep network structure, it's possible to tailor the optical paths and functionalities according to specific needs, enabling the creation of devices with arbitrary optical responses. This is achieved through a sophisticated design process that integrates simulation, optimization, and machine learning, allowing for the rapid prototyping and testing of photonic devices.
The architecture's modular nature not only facilitates the design of bespoke optical components but also significantly enhances the performance of photonic circuits. With the ability to design for minimal insertion loss and broad bandwidths, the deep photonic network platform can support ultra-broadband applications, making it an invaluable tool for advancing optical communication technologies.
This innovative architecture underscores the potential of photonic networks to revolutionize the field of integrated optics, offering a versatile platform for developing next-generation optical devices that are more efficient, faster, and capable of handling an unprecedented level of complexity.
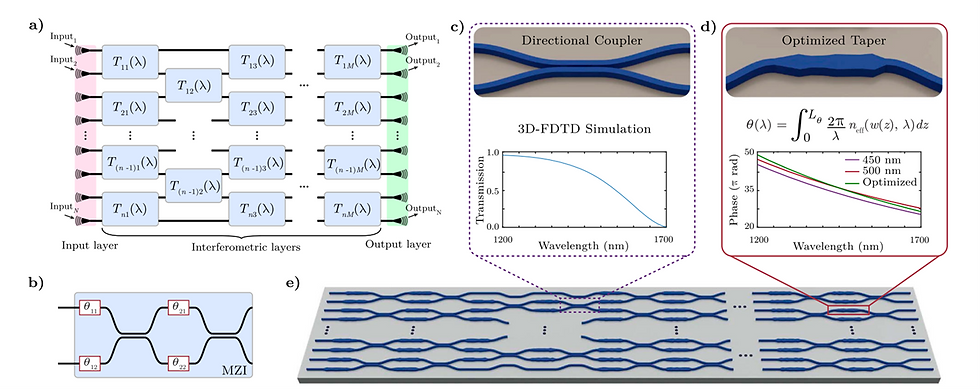
Simulation and Optimization Process
The simulation and optimization process within the deep photonic network architecture is a cornerstone that ensures the design of efficient and functional optical devices. This process leverages physics-informed machine learning algorithms to simulate the behavior of light within the network, taking into account the complex interactions between different optical elements. Through iterative simulations, the system can predict the optical response of the network with high accuracy.
Optimization plays a crucial role by refining the design parameters of the Mach-Zehnder Interferometers and other components to achieve the desired optical functionality. This involves adjusting the geometric and refractive properties of the network to minimize losses, enhance bandwidth, and ensure the targeted performance across a wide range of wavelengths.
This blend of simulation and optimization allows for the rapid prototyping of optical devices, significantly reducing the development time from concept to fabrication. By harnessing computational power and advanced algorithms, the process not only streamlines the creation of complex optical systems but also opens up new possibilities for innovation in photonic technologies.
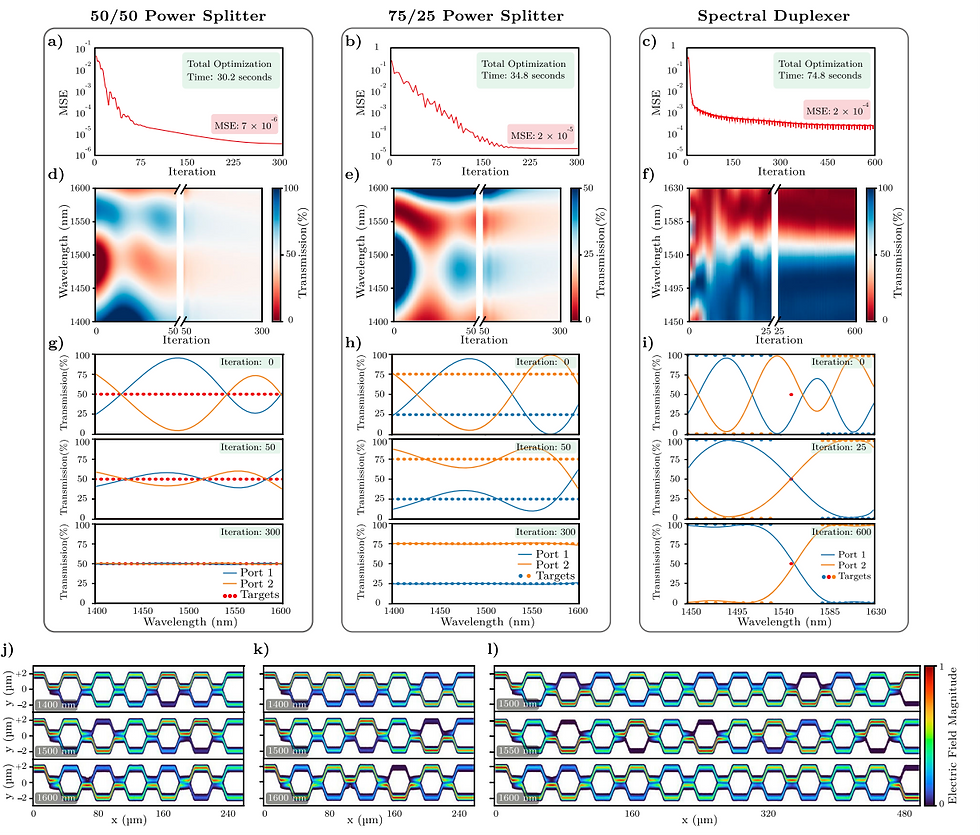
Experimental Demonstrations
The experimental demonstrations of the deep photonic network architecture validate its capability to design and fabricate optical devices with unprecedented functionality. Through a series of tests, devices such as ultra-broadband power splitters and spectral duplexers have been shown to perform with high efficiency, minimal insertion losses, and wide operational bandwidths. These demonstrations underscore the practicality and effectiveness of the network in addressing complex optical tasks, showcasing the seamless integration of design, simulation, and fabrication processes. The success of these experiments marks a significant milestone in the advancement of photonic integrated circuits, proving the viability of the deep photonic network platform for a wide range of applications in communications, computing, and sensing.
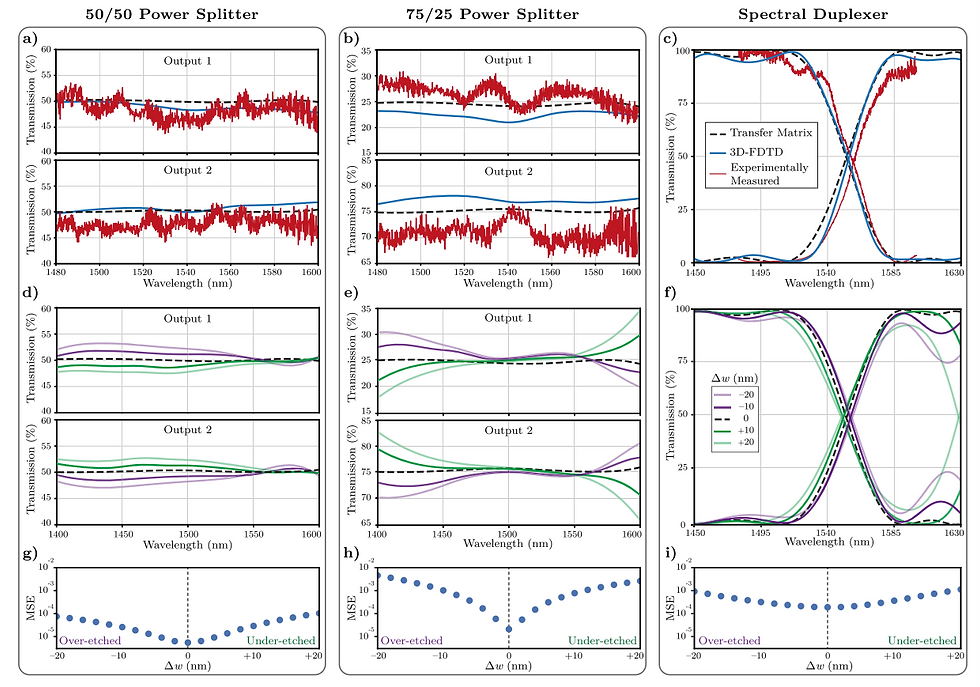
Scalability and Robustness
The scalability and robustness of the deep photonic network platform are among its most impressive features. This platform is designed to support the expansion of photonic integrated circuits, both in terms of complexity and size, without compromising on performance. The modular architecture allows for the addition of more components as needed, enabling the system to handle increasingly complex optical functions.
Furthermore, the robust design process, which integrates simulation and optimization, ensures that devices are not only effective in theory but also in practice. Experimental demonstrations have proven the platform's capability to produce devices with consistent performance, even when scaled up. This reliability is crucial for practical applications where precision and durability are paramount.
The platform's robustness against fabrication imperfections further demonstrates its suitability for commercial and industrial applications. This resilience ensures that the photonic devices can maintain high performance over time, making the deep photonic network architecture a solid foundation for the future of optical technologies.
Conclusion and Future Directions
The deep photonic network platform represents a significant advancement in photonic integrated circuits, offering a scalable, robust solution for designing and fabricating optical devices with unprecedented functionality. This architecture not only facilitates the rapid development of complex optical systems but also promises to revolutionize fields such as telecommunications, computing, and sensing. The successful experimental demonstrations underscore the platform's potential to meet the growing demands for high-speed, efficient optical communication systems.
Looking forward, the continued evolution of this technology is expected to unlock even more sophisticated applications, including in quantum computing and biomedical diagnostics. As research and development progress, the integration of more advanced machine learning models and fabrication techniques will further enhance the platform's capabilities, making photonic technologies more accessible and impactful across various sectors. The deep photonic network platform is poised to be at the forefront of the next wave of optical innovation.
Reference
[1] Najjar Amiri, A., Vit, A.D., Gorgulu, K. et al. Deep photonic network platform enabling arbitrary and broadband optical functionality. Nat Commun 15, 1432 (2024). https://doi.org/10.1038/s41467-024-45846-3
Comments