Introduction
Integrated optical phased arrays (OPAs) have become a crucial technology for enabling beam forming and steering in applications like light detection and ranging (LiDAR) and optical wireless communication (OWC). However, achieving precise phase control and managing phase errors in OPAs becomes more challenging as the number of channels scales up, due to factors such as electrical or thermal drift and fabrication errors. Conventional methods for OPA calibration typically rely on iterative optimization-based algorithms, such as genetic or hill-climbing methods, which often involve time-consuming and tedious procedures, particularly for scaled integrated OPAs.
To address these challenges, researchers at the Korea Advanced Institute of Science and Technology (KAIST) have proposed a novel phase control methodology leveraging a deep neural network (DNN) architecture tailored for a 64-channel integrated OPA. This approach aims to streamline the phase calibration process and improve the efficiency of beam steering control in these advanced optical systems.
Design and Fabrication
The designed layout of the 64-channel integrated silicon-based OPA chip is shown in Fig. 1(a). The OPA chip comprises an input grating coupler, a 6-stage multimode interferometer (MMI) splitter, 64 electro-optic (EO) phase shifters, and grating radiators, all optimized for the propagation of transverse electric (TE) mode at a wavelength of 1550 nm.

Fig. 1. (a) The designed layout of 64-channel optical phased array (OPA) and (b) fabricated OPA chip-on-board module with electrical wire-bonding.
Beam steering in the transversal direction is achieved by applying a forward bias voltage to each phase shifter, inducing phase changes through the plasma dispersion effect. Longitudinal beam steering is achieved using the thermo-optic (TO) effect, adopting an n-i-n heater on the grating radiator. The study focuses on phase control aspects related to transversal beam steering.
The OPA chip was fabricated at the National Nanofabrication Center (NNFC) on an 8-inch wafer with a 220 nm silicon-on-insulator (SOI) platform. The resulting chip is connected to a printed circuit board (PCB) through electrical wire bonding, as depicted in Fig. 1(b).
Data Gathering and Network Design
To train and evaluate the DNN model for OPA phase calibration, the researchers employed an experimental setup as shown in Fig. 2. The light source from a laser diode (LD) was coupled into the OPA module through a grating coupler. Voltage control for the 64 phase shifters on the OPA module was achieved using a digital-to-analog converter (DAC) board, controlled by custom LabVIEW software.
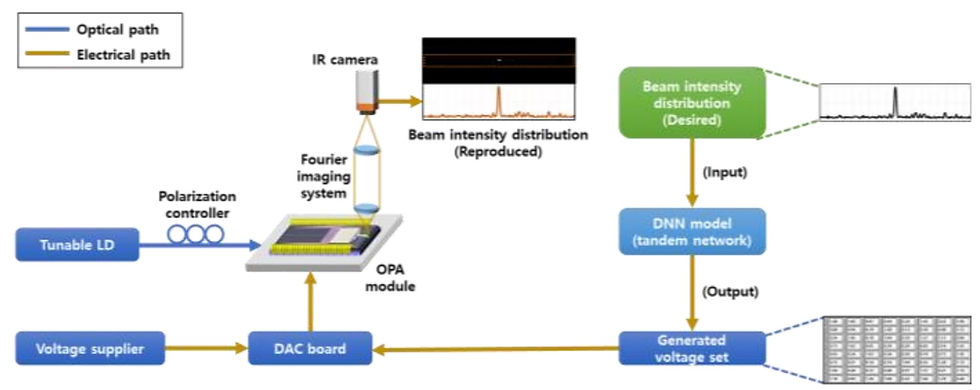
Fig. 2. Experimental setup for data collection to train the deep neural network (DNN) model for OPA phase calibration. This same setup was also used to assess the performance of the DNN model after the training.
Phase-tuned lights through individual phase shifters were eventually emitted from the radiator, generating far-field beam patterns. A Fourier imaging system was then employed to capture the far-field beam distribution, and the corresponding 1-D beam distribution was derived from the resulting 2-D image. The collected data was utilized to train the DNN model, which could reproduce the desired beam intensity distribution by inputting the generated voltage settings into the OPA.
To address the complexity of phase control in a 64-channel OPA and non-uniqueness issues, the researchers adopted a tandem network architecture, as shown in Fig. 3. This architecture streamlines the training steps, ensuring a one-to-one mapping between the desired beam distribution and voltage set.
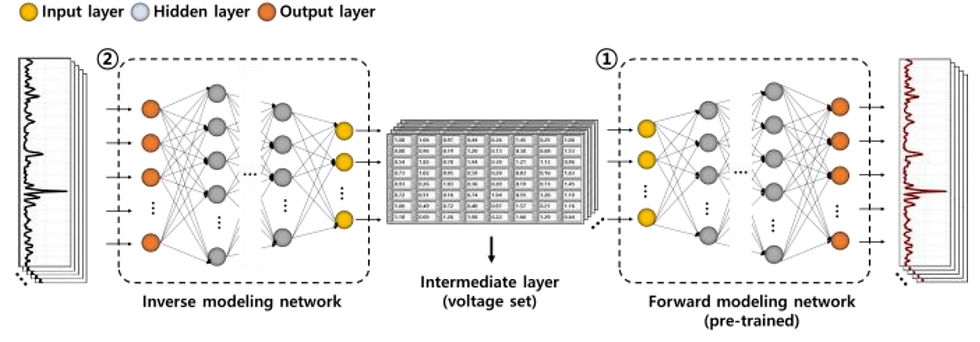
Fig. 3. A schematic diagram of the proposed tandem network model for OPA phase control. The model consists of two interconnected networks: the pre-trained forward modeling network and the inverse modeling network.
The training procedure involved initially training the forward modeling network, then fixing its weights, followed by training the inverse modeling network. Hyperparameter tuning and Mean Square Error (MSE) loss function were applied to both networks, minimizing deviation during training.
Experimental Results
The researchers assessed the beam prediction performance of their trained DNN model with 20 evaluation datasets searched by the hill-climbing algorithm (HA) as references, observing consistent results in 16 out of 20 cases.
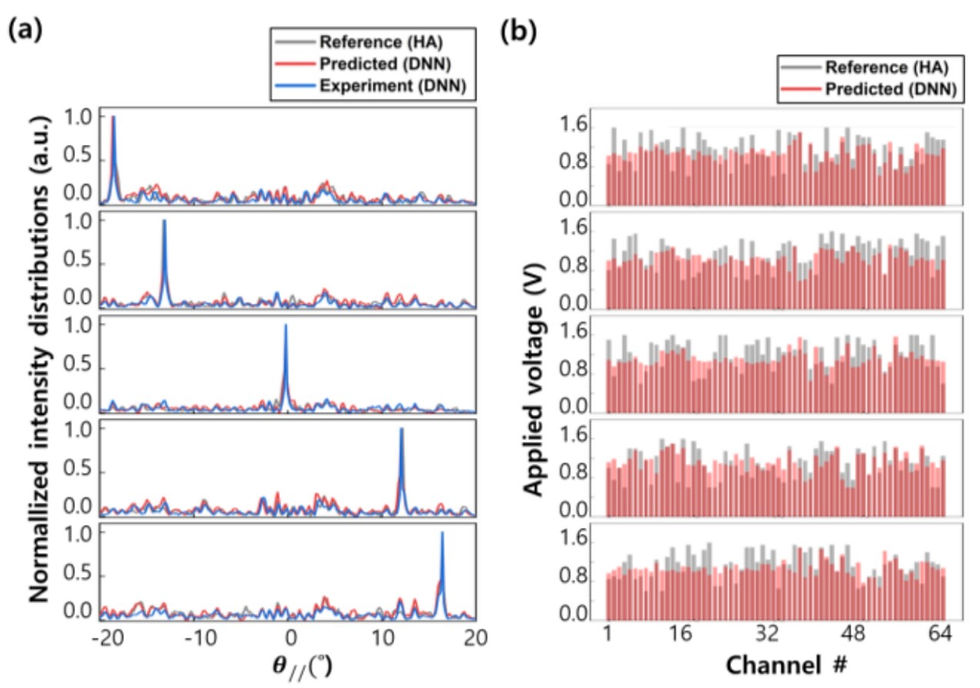
Figure 4(a) shows 5 representative normalized beam distributions in different directions, comparing three scenarios: the reference beams calibrated by the HA (grey), the predicted beam intensity distribution from the trained DNN (red), and the experimental beam intensity distribution obtained using the predicted voltage set from the trained DNN (blue).
Additionally, Fig. 4(b) presents a side-by-side comparison of the voltage sets extracted from the trained network's intermediate layer and the voltage sets obtained using HA for 5 different far-field beam intensity distributions.
The normalized experimental beam distributions resulting from the predicted voltage set exhibited an average side-mode-suppression-ratio (SMSR) of 7.05 dB across 5 distinct beam distributions, slightly surpassing the 6.88 dB attained in the network's simulated results. This level of SMSR closely aligns with the average SMSR of 7.11 dB observed in the normalized beam distributions achieved using the hill-climbing algorithm (HA).
Although there was a slight reduction in peak intensity of 0.84 dB on average, the experimental beam distributions closely matched the quality of the HA-calibrated beams, demonstrating the DNN's ability to successfully reproduce the desired beam distribution.
Conclusion
The researchers at KAIST have demonstrated a deep learning-leveraged phase calibration methodology for OPA beamforming. While minor performance degradations were noted compared to conventional hill-climbing algorithms, their approach simplifies calibration for multiple beam points, offering promise for optical systems and photonic applications. This novel methodology leverages the power of deep learning to streamline and enhance the phase calibration process in advanced integrated optical phased arrays, paving the way for more efficient and precise beam steering control in emerging technologies like LiDAR and optical wireless communication.
Reference
[1] J.-Y. Kim, J. Kim, J. Yoon, and H. Kurt, "Deep Learning-Driven Phase Calibration Methodology for Enhanced Beamforming in Integrated Optical Phased Array," School of Electrical Engineering, Korea Advanced Institute of Science & Technology (KAIST), Daejeon, Republic of Korea, 2024, pp. 1-6, doi: 979-8-3503-9404-7/24/$31.00 ©2024 IEEE.
Comentários